注釈
Go to the end をクリックすると完全なサンプルコードをダウンロードできます.
ボリュームレンダリング#
pyvista.ImageData
または3 D NumPy配列のようなボリュームレンダリングの均一メッシュタイプ.
これはまた pyvista.ImageData.extract_subset()
フィルタを使用して pyvista.ImageData
から関心領域 (VOI) を抽出する方法を探求します.
from __future__ import annotations
import numpy as np
import pyvista as pv
from pyvista import examples
# Download a volumetric dataset
vol = examples.download_knee_full()
vol
シンプルボリュームレンダー#
# A nice camera position
cpos = [(-381.74, -46.02, 216.54), (74.8305, 89.2905, 100.0), (0.23, 0.072, 0.97)]
vol.plot(volume=True, cmap='bone', cpos=cpos)
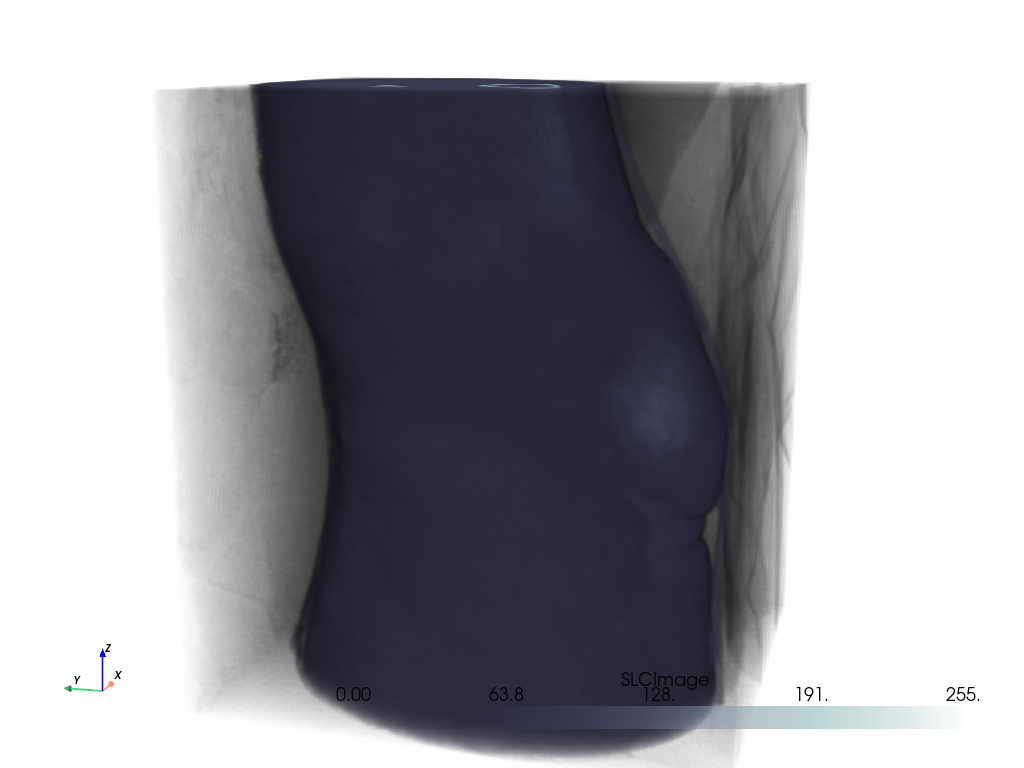
不透明度マッピング#
または,以下のように pyvista.Plotter.add_volume()
メソッドを使用します.ここでは,シグモイドへのデフォルト以外の不透明度マッピングを使用することに注意してください.
pl = pv.Plotter()
pl.add_volume(vol, cmap='bone', opacity='sigmoid')
pl.camera_position = cpos
pl.show()
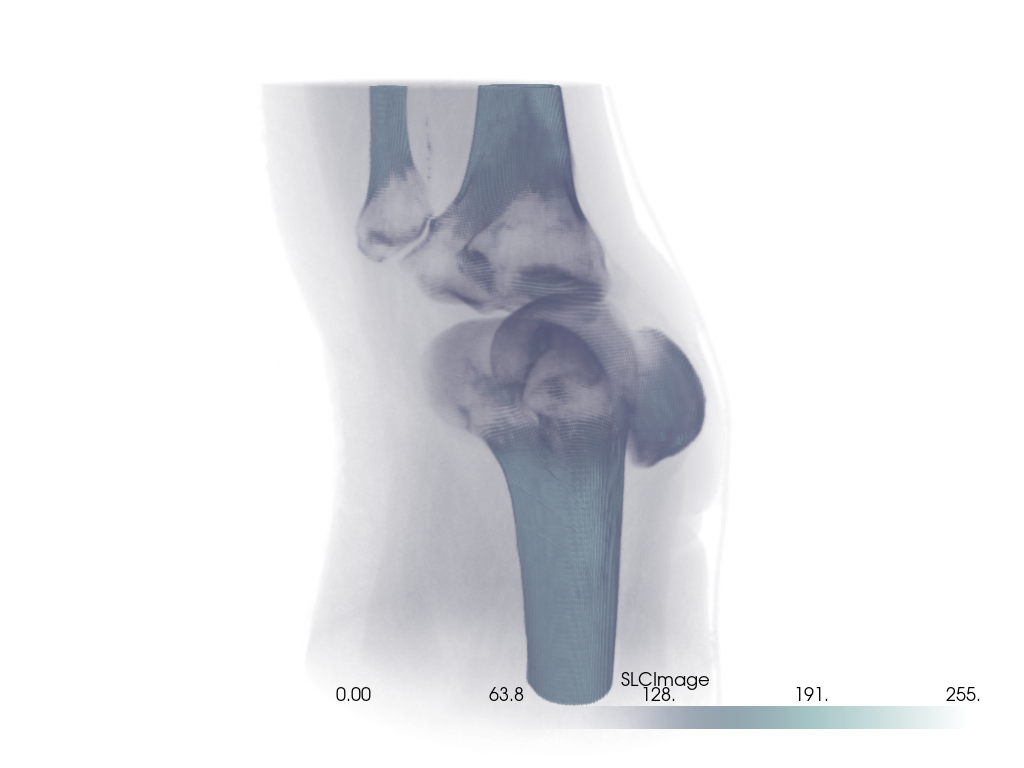
カスタム不透明度マッピングを使用することもできます.
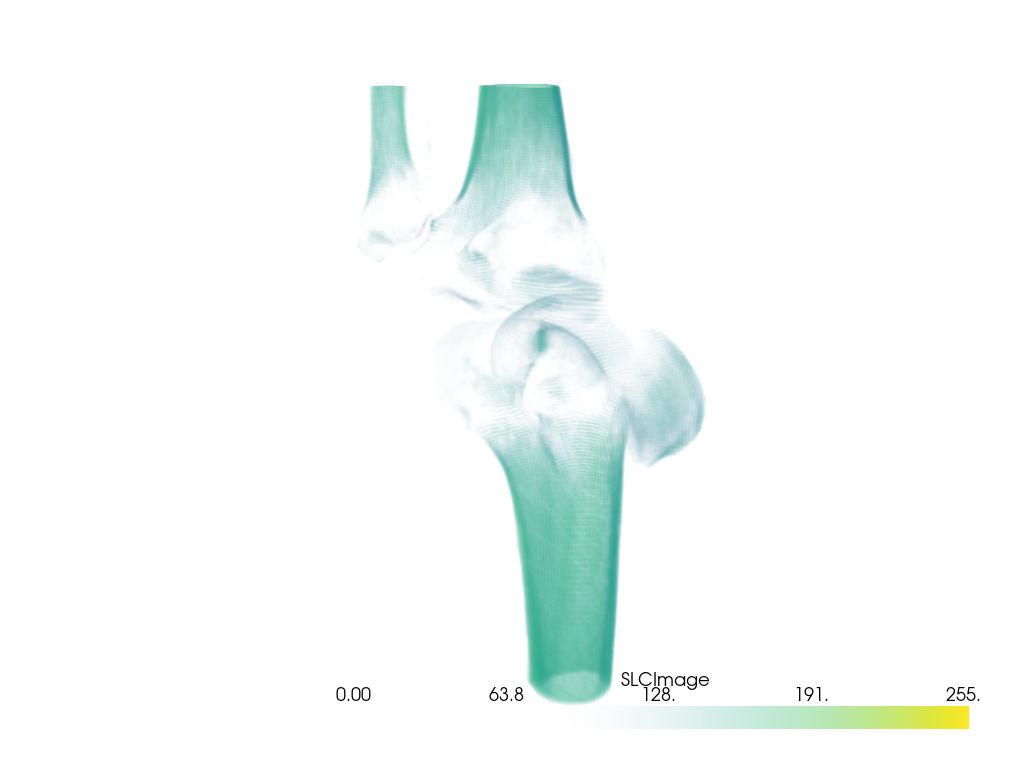
shade
オプションを使用してボリュームレンダリングを行う場合は,シェーディングテクニックを使用することもできます.
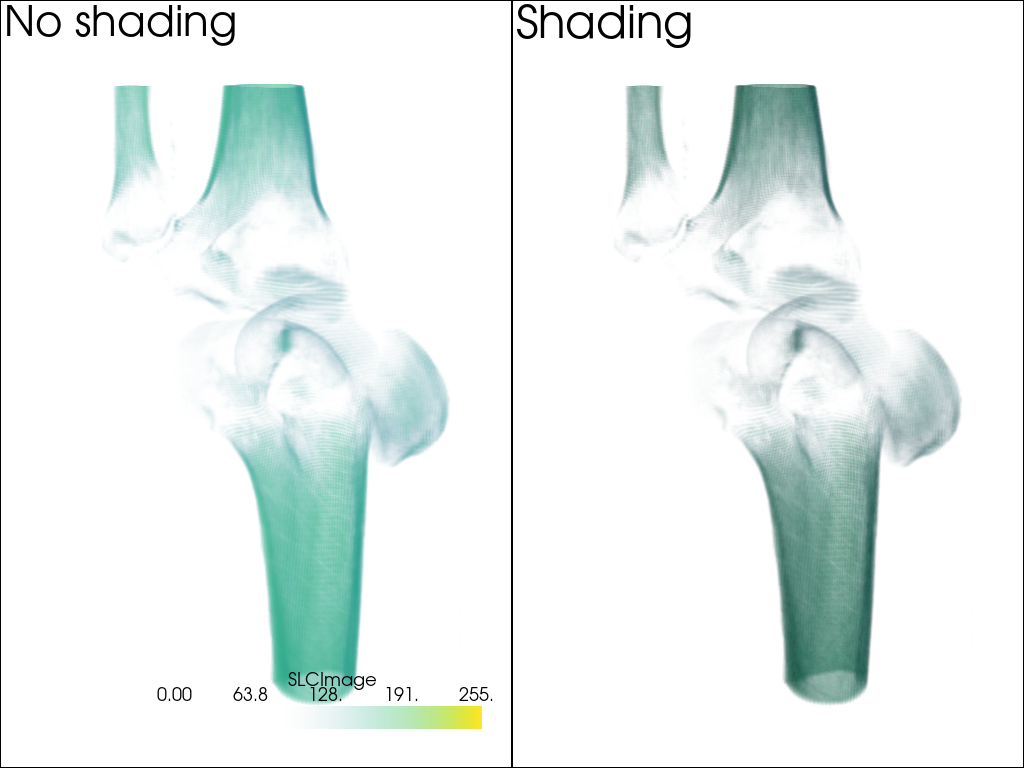
かっこいいボリュームの例#
ここでは,クールなボリュームレンダリングの例をいくつか紹介します.
ヘッドデータセット#
head = examples.download_head()
pl = pv.Plotter()
pl.add_volume(head, cmap='cool', opacity='sigmoid_6', show_scalar_bar=False)
pl.camera_position = [(-228.0, -418.0, -158.0), (94.0, 122.0, 82.0), (-0.2, -0.3, 0.9)]
pl.camera.zoom(1.5)
pl.show()
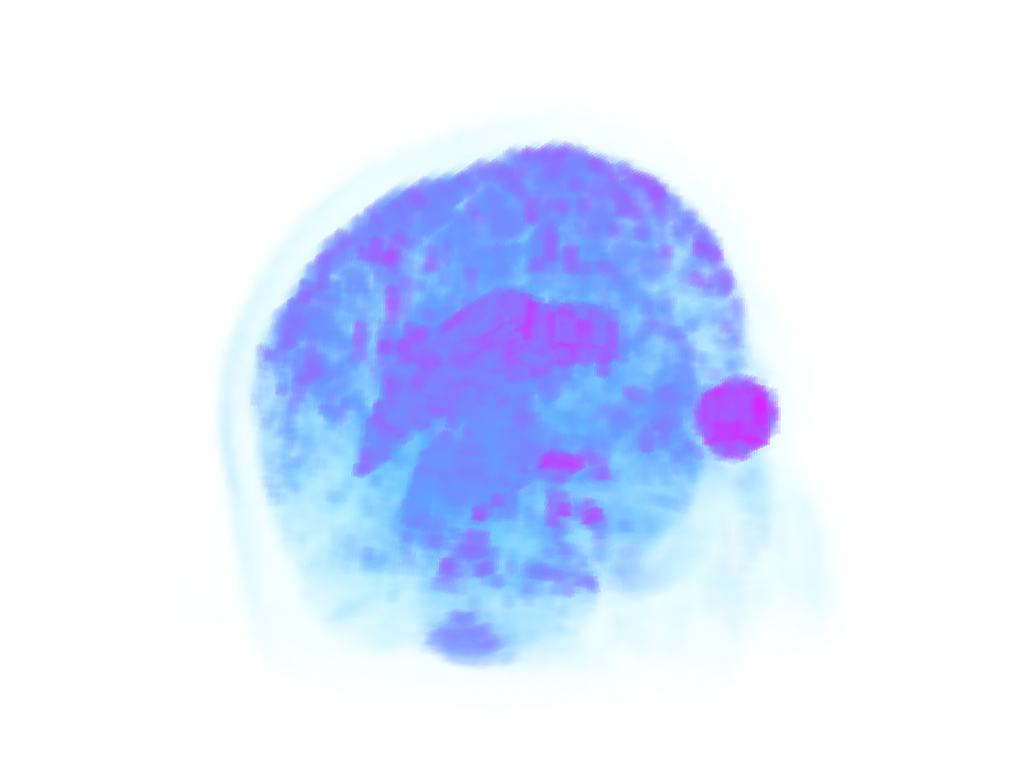
ボルト・ナット式マルチブロックデータセット#
注釈
ここでは,より魅力的なプロットを作成するために,個々のセルのスカラーを滑らかにするために,補間を 'linear'
に設定したことを確認してください. bolt_nut
は pyvista.MultiBlock
というデータセットなので, add_volume
が返すアクターは 2 つになります.
bolt_nut = examples.download_bolt_nut()
pl = pv.Plotter()
actors = pl.add_volume(bolt_nut, cmap='coolwarm', opacity='sigmoid_5', show_scalar_bar=False)
actors[0].prop.interpolation_type = 'linear'
actors[1].prop.interpolation_type = 'linear'
pl.camera_position = [(127.4, -68.3, 88.2), (30.3, 54.3, 26.0), (-0.25, 0.28, 0.93)]
cpos = pl.show(return_cpos=True)
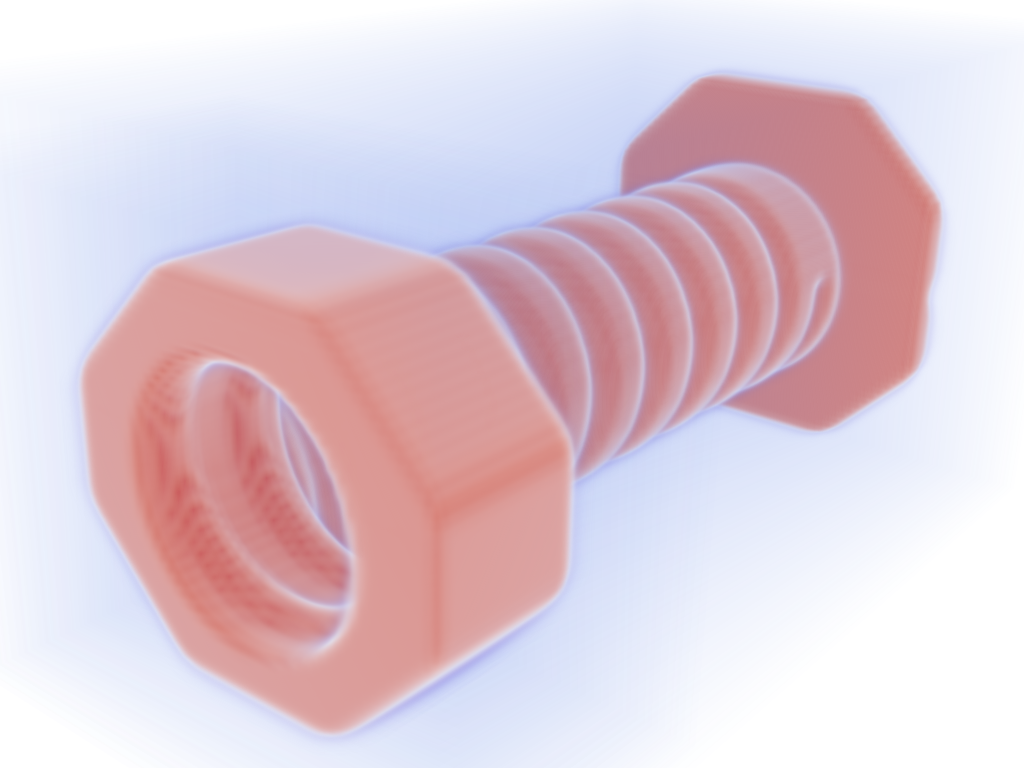
カエルデータセット#
frog = examples.download_frog()
pl = pv.Plotter()
pl.add_volume(frog, cmap='viridis', opacity='sigmoid_6', show_scalar_bar=False)
pl.camera_position = [(929.0, 1067.0, -278.9), (249.5, 234.5, 101.25), (-0.2048, -0.2632, -0.9427)]
pl.camera.zoom(1.5)
pl.show()
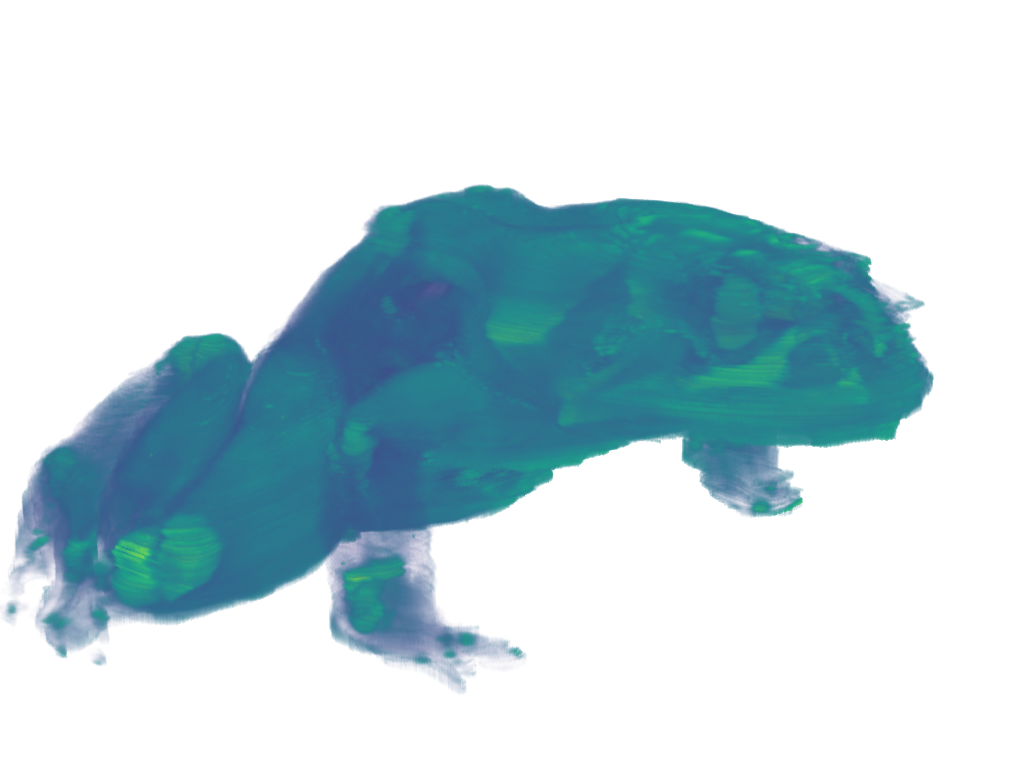
VOIの抽出#
pyvista.ImageDataFilters.extract_subset()
フィルタを使用して,ボリュームレンダリングの対象となるボリューム/サブセットボリュームを抽出します.これは,特に大きなボリュームを処理し,特定の領域のみをボリュームレンダーする場合に理想的です.
# Load a particularly large volume
large_vol = examples.download_damavand_volcano()
large_vol
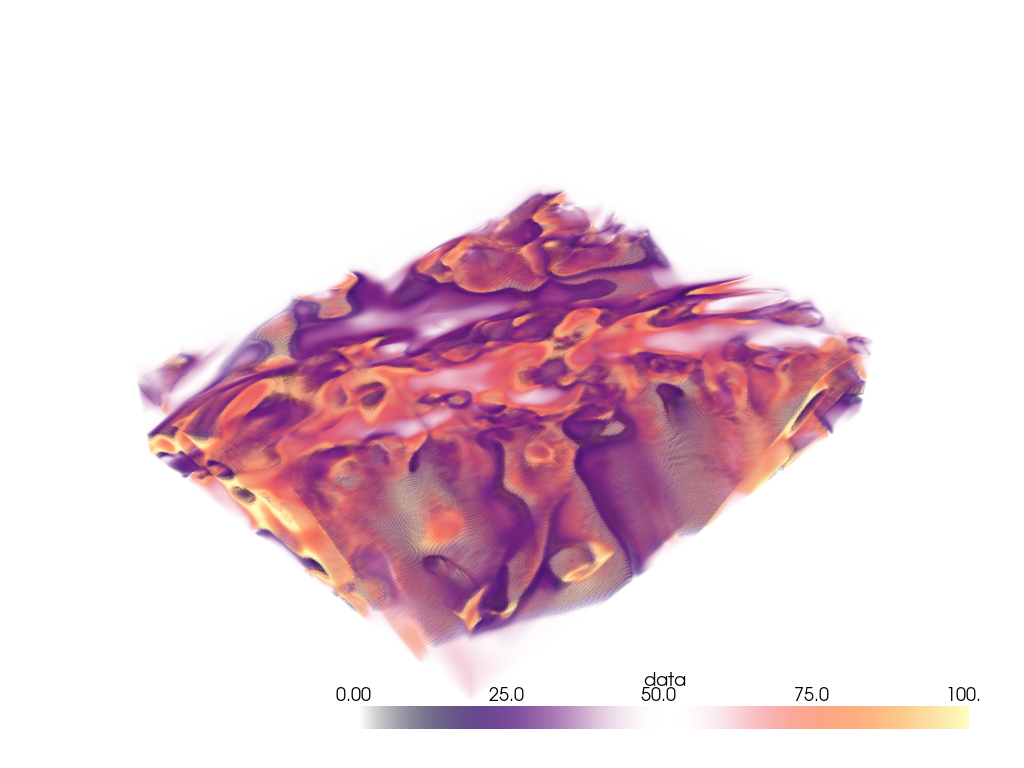
わあ,すごいボリュームだ.全体をボリュームレンダリングしたくはないでしょう.火山の下の興味深い地域を抽出してみましょう.
抽出する領域は,x軸上の節点175と200の間,y軸上の節点105と132の間,およびz軸上の節点98と170の間になります.
voi = large_vol.extract_subset([175, 200, 105, 132, 98, 170])
pl = pv.Plotter()
pl.add_mesh(large_vol.outline(), color='k')
pl.add_mesh(voi, cmap='magma')
pl.show()
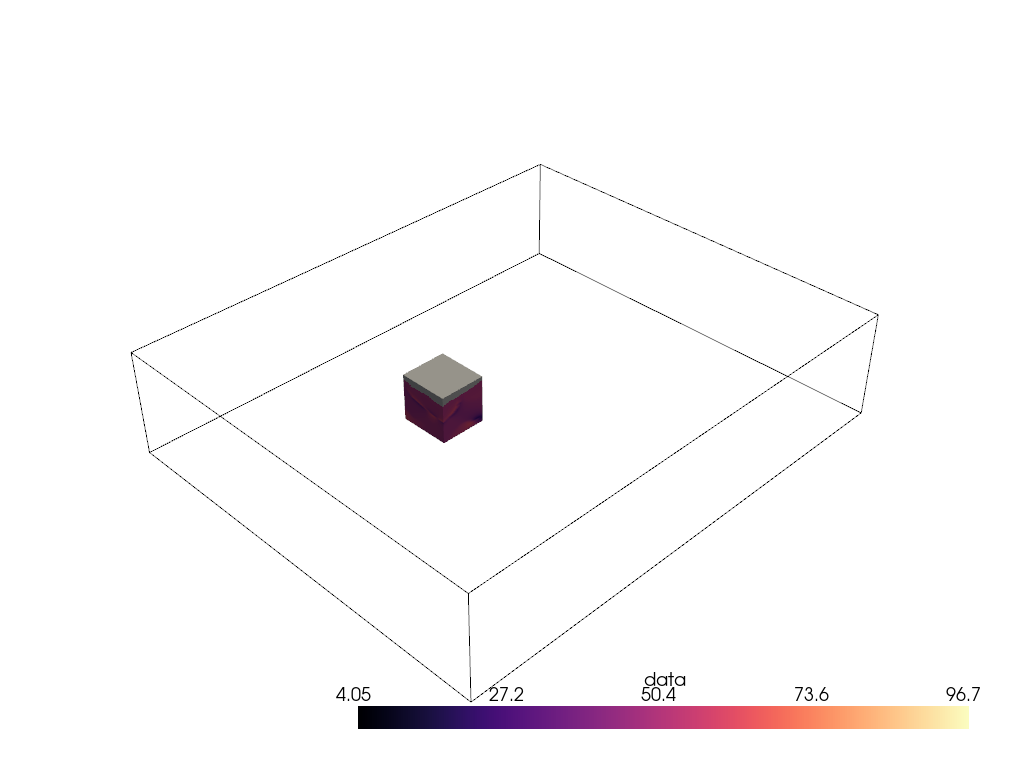
ああ,だいぶよくなりました.次に,その対象領域をボリュームレンダリングします.
pl = pv.Plotter()
pl.add_volume(voi, cmap='magma', clim=clim, opacity=opacity, opacity_unit_distance=2000)
pl.camera_position = [
(531554.5542909054, 3944331.800171338, 26563.04809259223),
(599088.1433822059, 3982089.287834022, -11965.14728669936),
(0.3738545892415734, 0.244312810377319, 0.8947312427698892),
]
pl.show()
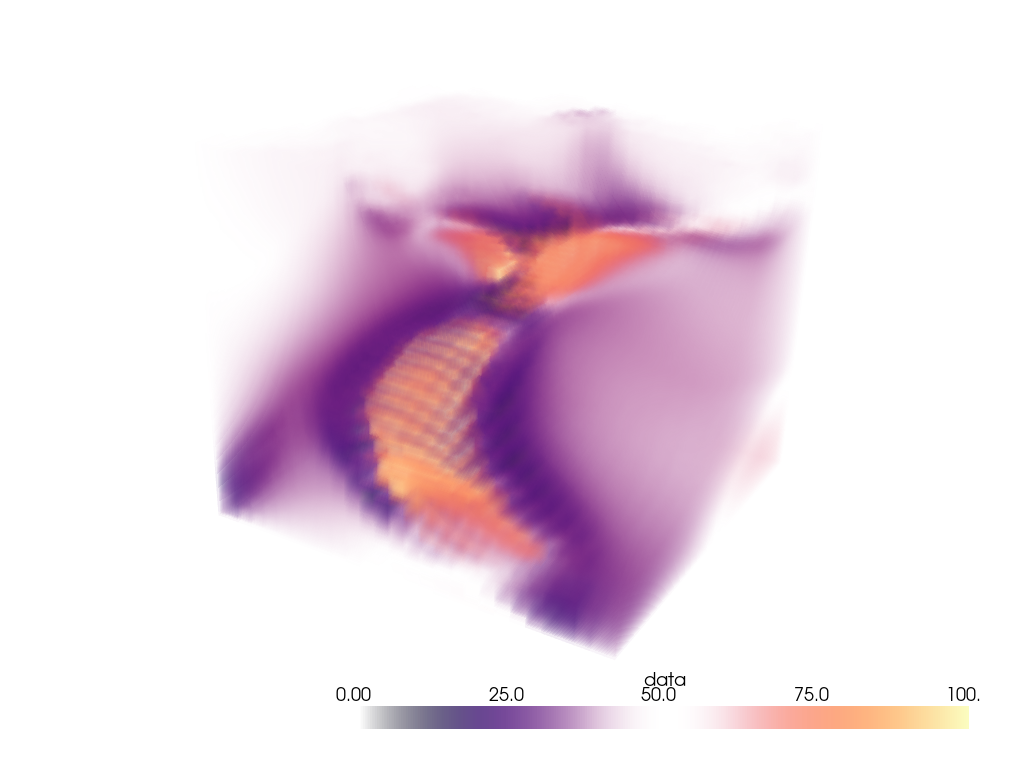
Volume With Segmentation Mask#
Visualize a medical image with a corresponding binary segmentation mask.
For this example, we use download_whole_body_ct_male()
though download_whole_body_ct_female()
, or any
other dataset with a corresponding label or mask may be used.
Load the dataset and get the ct image and a mask image. Here, a mask of the heart is used.
dataset = examples.download_whole_body_ct_male()
ct_image = dataset['ct']
heart_mask = dataset['segmentations']['heart']
Use the segmentation mask to isolate the heart in the CT image.
Initialize a new array and image with CT background values. Here, we set the scalar
values to -1000
which typically corresponds to air (low density).
heart_array = np.full_like(ct_image.active_scalars, -1000)
Extract the intensities for the heart segment. We use heart mask's array to mask the CT image to only extract the intensities of interest.
ct_image_array = ct_image.active_scalars
heart_mask_array = heart_mask.active_scalars
heart_array[heart_mask_array == True] = ct_image_array[heart_mask_array == True] # noqa: E712
Add the masked array to the CT image as a new set of scalar values.
ct_image['heart'] = heart_array
Create the plot.
For the CT image, the opacity is set to a sigmoid function to show the
subject's skeleton. Since different images have different intensity
distributions, you may need to experiment with different sigmoid functions.
See add_volume()
for details.
pl = pv.Plotter()
# Add the CT image.
pl.add_volume(
ct_image,
scalars='NIFTI',
cmap='bone',
opacity='sigmoid_15',
show_scalar_bar=False,
)
# Add masked CT image of the heart and use a contrasting color map.
_ = pl.add_volume(
ct_image,
scalars='heart',
cmap='gist_heat',
opacity='linear',
opacity_unit_distance=np.mean(ct_image.spacing),
)
# Orient the camera to provide a latero-anterior view.
pl.view_yz()
pl.camera.azimuth = 70
pl.camera.up = (0, 0, 1)
pl.camera.zoom(1.5)
pl.show()
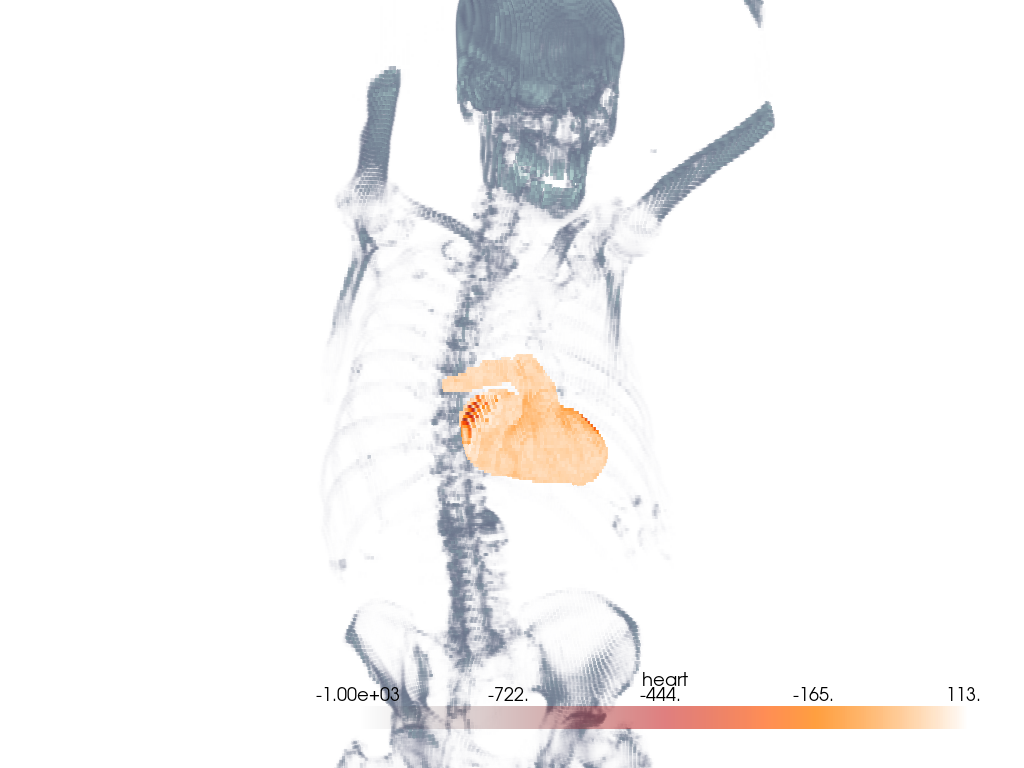
Total running time of the script: (0 minutes 41.397 seconds)